Initial Analysis of Our Accurate Forecasting of 2020 Taiwan General Election with ABM
At 10 am (Beijing time) on January 9, 2020 (two days before the actual voting in Taiwan), our research team at the Center for Commplex Decision Analysis (directed by by the frist author) of Fudan University, Shanghai, China, released our forecast results for the upcoming Taiwanese presidential election on January 11, 2020.
English version link:
https://www.linkedin.com/pulse/2020-taiwan-elections-forecasted-computer-simulation-shiping-tang-dr/
[Our forecasting is based on agent-based modeling (ABM), with zero reliance on opinion polls. We have been developing this method since early 2015. For the releases of our earlier forecasting efforts, which have also been fairly accurate, see the links at the end of this article (Appendix).]
For the 2020 Taiwan election, our two sets of forecasting models predict that Green Camp (Tsai Ing-wen) will win the election with 56.86% or 56.42% of the popular votes, on average. The first set of models (group A) models) predict that the Gree Camp wil receive anywhere between 55.17% (lowest) and 59.48% (highest) of the total votes. The second set of models (group B models) predict that the Gree Camp wil receive anywhere between 54.06% (lowest) and 59.74% (highest) of the total votes. [Apparently, the first set of models have a smaller interval.]
By mid-night of January 11, 2020, final results of the Taiwan 2020 elections came in. Our forecasted results are very close to the final actual election result (Tsai won 57.13% of the votes), and the difference is only 0.27% for models in Group A and 0.71% for models in Group B. [A key difference between group A models and group B models is that among the various variables in our models, the former contains employment in which sector (agriculture, manufacturing, or service) whereas the latter replaces it with religious beliefs.]
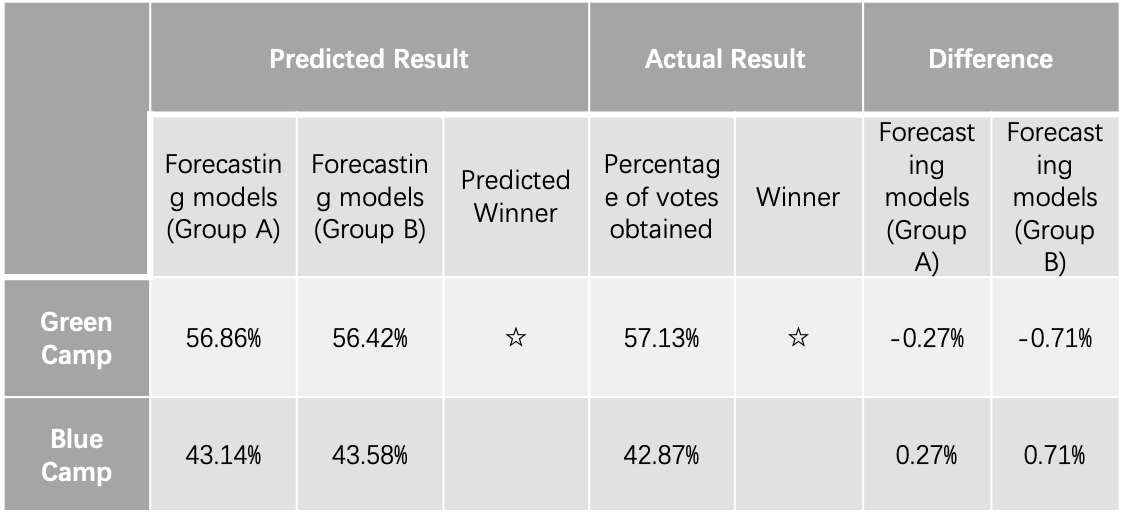
This forecasting success follows our team’s accurate forecasting of the Taiwanese general election in 2016, the mid-term US Senate elections in West Virginia and Missouri in 2018, and the mayoral elections of Taipei, New Taipei and Taoyuan in Taiwan in 2018. For more information, please visit our official website:
www.ccda.fudan.edu.cn
The forecasting result is based on computer simulation and does not depend on any poll data. This forecasting research mainly uses the method of Agent Based Modeling (ABM). ABM allows us to to simulate emergemt outcomes from the actions and interactions of agents (e.g. voters) within a complex system. Agents with an ABM system are endowed with unique attributes and our voting behavior is rule based. The prominent feature of this forecasting method is that it operates mostly on objective data and uses agent-based computational modeling, instead of mostly relying on opinion surveys, experts’ individual judgment, and fragmented news information as most existing election forecasting methods do.
The forecasting models include multiple variables that may affect the election result. Prior to the forecasting, the team conducted long-term, large-scale, multi-dimensional data collection, and finally constructed a dataset that covers voters’ demography, previous voting results, economic and social condition, candidate characteristics, critical events, etc. Our whole dataset contains more than 50 variables.
In the process of simulation, the team strives to (1) conduct variable selection and model construction under the guidance of election theories in political science; (2) combine individual-level data of voters and candidates with structural-level data of economic and social development; (3) combine long-term steady effects and short-term fluctuation effects, in an attempt to forecast the election more accurately through standardized models, rigorous procedures, and computational algorithm.
We are now drafting a full paper that will provide more technical details about our approach.
Appendix: Earlier relases of our previous efforts
Four years ago, on Jan. 05, 2016 (Chinese version) and Jan. 10, 2016 (English version), that is, before the election in Taiwan was started that year, Tang’s team released our forecasting of this election. That prediction was our first attempt of election forecasting with our new approach. For details, see:
Chinese Version:
http://www.ccda.fudan.edu.cn/index.php?c=article&id=75
English Version:
https://china.ucsd.edu/_files/01112016_Taiwan-election.pdf;
https://chinafocus.ucsd.edu/2016/01/10/taiwan-election-results-predicted-in-computer-simulation/
On Nov. 04, 2018, Our team released our forecasting of US Senate Elections in two states (West Virginia and Missouri) before the actual voting of the two elections. That forecasting was our second attempt of election forecasting with our new approach. For details, see:
Chinese Version:
http://blog.sina.com.cn/s/blog_744a73490102y7bd.html
http://www.ccda.fudan.edu.cn/index.php?c=article&id=80
English Version:
http://www.ccda.fudan.edu.cn/index.php?c=article&id=81
https://www.linkedin.com/pulse/united-states-senate-elections-west-virginia-missouri-tang-dr/?trackingId=shAzPfa2GP9KRu%2BbwvnIyQ%3D%3D
Results compared:
Chinese Version: http://www.sirpa.fudan.edu.cn/?p=12377
Brief English version:
https://www.linkedin.com/pulse/prelimary-assessment-our-forecasting-excellent-perhaps-tangdr/?trackingId=vmFPQdRhrHHJhTrkX%2Bh%2F3Q%3D%3D
On Nov. 22, 2018, Tang’s team released our forecasting of Taiwan local elections (Taipei, New Taipei, and Taoyuan) before the elections were started that year. That prediction was our third attempt of election forecasting with our new approach. For details, see:
Chinese Version:
http://www.ccda.fudan.edu.cn/index.php?c=article&id=83
English Version:
http://www.ccda.fudan.edu.cn/index.php?c=article&id=84
Results compared:
Chinese Version:
http://www.ccda.fudan.edu.cn/index.php?c=article&id=85